Representative Quotations
Introduction
Abstract
Brexit was the withdrawal of the United Kingdom from the European Union. The United Kingdom is the first and so far the only member state to have left the EU, after 47 years of having been a part of the union. It is an event that has made people very vocal and different opinions have been shared, particularly in the media. In this project, we want to put this event under the microscope and investigate several aspects of it. First, we want to do a descriptive analysis: When did we start mentioning Brexit, which words are used the most in our database, which are the main authors of the quotes, which media have relayed the information the most, etc.
Datasets
We applied our data analysis on the following three datasets
- Quotebank
- Speaker Attributes
- Country code top-level domain(ccTLD)
- ISO-3166-Countries-with-Regional-Codes
Quotebank is a dataset of 178 million unique, speaker-attributed quotations that were extracted from 196 million English news articles crawled from over 377 thousand web domains between August 2008 and April 2020. Our analysis only focus on Brexit related quotations, we only used datasets from 2015 to 2020 in Quotebank. To filter quotations related to Brexit, we firstly filtered any quoation contains both "EU" and "UK" (combinations of full names and abbreviations) or any quotation contains "Brexit". Then we filtered previous selected quotations for a second round, we only kept previous selected quotations which contain any key word in ['brexit','leave campaign and remain campaign','no deal', 'transition period', 'leave', 'withdral', 'referendum', 'split from'].
Speaker attributes dataset is provided by ADA teaching team which which extract some information about speakers such as nationality, occupation, party etc. from the Wikidata knowledge base.
To extract the country of the domain for further analysis, we used beautifulsoup through Country code top-level domain(ccTLD) page
For map plotting, we need the ISO-3166 alpha‑3 codes for all countries. So we extract part of csv from the ISO-3166-Countries-with-Regional-Codes/all.csv file.
Methods
-
Data Collection
-
Sentiment Analysis
-
LDA Topic Modeling
-
Spectral Clustering
To merge quotebank with the additional datasets, we have filtered those quotations related to Brexit by identifying possible keywords related to Brexit as stated in Datasets section. We have already combined quotations with speaker attributes by joining with QIDs. We also scrapped the web page to get the domain countries using beautiful soup for future analysis.
We then carried out a sentiment analysis, for that we used the results of three packages: Textblob, SentimentIntensityAnalyze and Flair. We have then taken the median of the three resulting polarities. To subsequently decide on the thresholds to choose for classifying the emotions (Positive, Negative, or Neutral), we manually labeled 100 quotes and selected the thresholds accordingly. It is critical to note that the resulting emotion does not reflect the direct view or perception of the Brexit, i.e. pro or con. The resulting emotion is based on the tone of the quote. As an example (fabricated), the two quotes: "Brexit is crucial, staying in the union would be a catastrophe, a suicide" and "Staying in the union is crucial, leaving would be a catastrophe, a suicide" will both be assigned a negative emotion by design even though they stand for two different opinions.
Using the n-garms and LDA topic modeling, we want to identify the top topics to find some of the reasons that motivated their opinions.x. We also want to visualize the change of predominant opinions and the central topics and points mentioned.
Spectral clustering was applied for speakers and for different subclasses of occupation over time. We first encoded the category features-nationality, polarity, and occupation-using two methods: hot encoding and target encoding. We then used the T-SNE method to compress the features and generate tsne_x,tesn_y so that they could be plotted in 2D or 3D graphs. We obtained some plots and results, but we will leave the final interpretation and more advanced implementation of the T-SNE approach to be done as a future improvement of our T-SNE method. You can find the first results, uncommented, of the cluster analysis at the end of this notebook
I. Media's Coverage
All Quotes in Quotebank
Brexit-related Quotes for our analysis
Speakers related to Brexit
The original Quotebanks dataset contains 115,584,257 records. As described in the DataPreProcessing notebook, we initially extracted quotes that were solely about Brexit, the United Kingdom and/or the European Union. We then proceeded with filtering these records to consider only those containing one of the words from the following list: leave campaign and remain campaign, no deal, transition period, leave, withdral, referendum and split from as Brexit-related quotes. As a result, we ended up with 101,878 Brexit-related citations and 14,456 speakers who own Brexit-related quotations for more in-depth analysis.
I.1 When did the media start to mention Brexit?
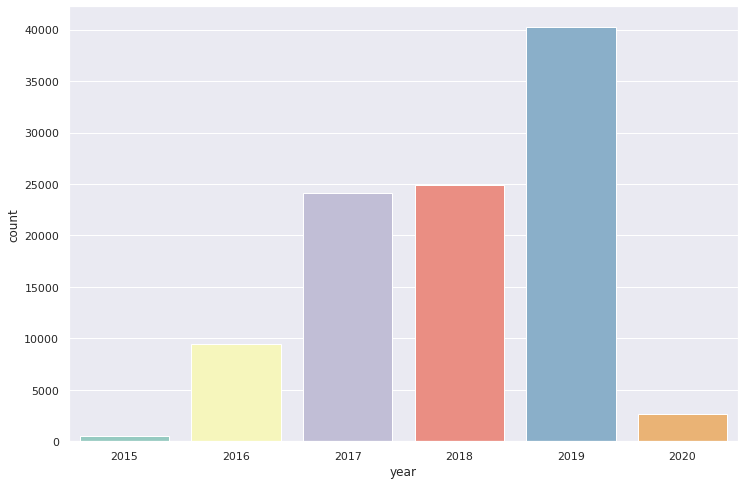
Always considering the filtered Quotebank data, the graph above represents the number of citations related to the Brexit over the years. We first notice that before 2015, only a few hundred citations were related to Brexit (about 0.5% of the Brexit related quotes). This switches in 2016 - the year when the Brexit was voted- and we can see that until 2019, the number of citations will keep increasing until waiting for its peak, reaching 39.51% of the total Brexit related data. Yet in 2020, we witness a sharp drop. We suggest that this is because most news stories were relaying information about the coronavirus at that time. Another theory would be that the data was not completely extracted from year 2020. In order to further analyze this trend over the years, we look at what happens each year while looking at the dates that marked the Brexit.
I.2 How did the number of quotations change over time?
- June 23, 2016 - The UK votes to leave in a referendum. The "Leave" camp, which favors the UK's exit from the EU, won with 51.9%, compared to 48.1% for the pro-EU "Remain" camp.
- July 13, 2016 - Theresa May becomes Prime Minister following the resignation of David Cameroon.
- March 29, 2017 - Warning: two-year countdown to the UK's exit from the European Union. The UK is then negotiating an exit agreement.
- December 8, 2017 - Birth of the backstop: tensions form on Irish borders. In the event of an exit without an agreement of the United Kingdom from the EU, the 500 kilometers that separate the British province of Northern Ireland from the Republic of Ireland could become a physical border again. As London has decided to leave the single market and the customs union, which are synonymous with freedom of movement and common standards and customs duties, border controls will be necessary. However, this return to a border between the two countries would weaken the Good Friday Peace Agreement, which ended, in 1998, thirty years of armed conflict between nationalists and unionists in Northern Ireland.
- November 25, 2018- After a period of calm, the subject of the Backstop resurfaces a second time.
- June 24, 2019 - May takes a bow: Theresa May submits her resignation, following her failure to pass her plan to withdraw from the European Union.
- July 24, 2019- the Johnson era begins: Boris Johnson is elected leader of the Conservative Party the following year, he succeeds Theresa May as Prime Minister, promising a rapid exit of the United Kingdom from the European Union.
- 28 August 2019- Parliament put on ice for 5 weeks: In August, reports emerged that the new PM had asked the Queen to suspend Parliament for five weeks in the run-up to 31 October.
- 2 October 2019 - Johnson sets out his ‘reasonable compromise’ Brexit deal: By early October, the PM had made a formal proposal to the EU setting out his alternative to the Irish backstop. He claimed his plan was “entirely compatible with maintaining an open border in Northern Ireland”, unlike the “bridge to nowhere” backstop.
- 19 October 2019 - the showdown: Parliament hosted a special session for MPs on Saturday 19 October - less than two weeks before the Halloween Brexit deadline. It was the fifth time Parliament sat on a Saturday for 80 years, with the previous occasions including include the day before the outbreak of the Second World War, the Suez Crisis in 1956 and the Falklands War in 1982, says The Guardian. Johnson was legally obliged by the Benn Act to send a letter to the EU on that date requesting a three-month Brexit extension after Parliament refused to pass his deal.
- 31 January 2020 – departure day: Having won the majority he so desired in December, Johnson passes his withdrawal agreement, paving the way for the UK to leave the EU on 31 January.
On the graph, we can see that before the vote on June 23, 2016, the number of citations was very limited. The number started to increase until July 13, 2016, the day Theresa May became PM, before dropping again and entering a stationary phase. We anticipated seeing more dramatic variations in the number of quotes tying in with these specific dates, though we must keep in mind that the plot only considers data from Quotebank, which may not represent what is actually shared by all media outlets.
II - Media views towards Brexit:
II.1 Which media have relayed the information the most?
Among the top medias we see:
- The Daily Express (here Express) and its sister paper - the Sunday Express are daily national middle-market and conservative tabloid newspapers in the United Kingdom.
- The Belfast Telegraph is a daily newspaper published in Belfast, Northern Ireland, by Independent News & Media.
- The Herald is a Scottish broadsheet newspaper founded in 1783. The Herald is the longest running national newspaper in the world and is the eighth oldest daily paper in the world.
- The Politico is a political journalism company based in Arlington County, Virginia, that covers politics and policy in the United States and internationally. It primarily distributes content online but also with printed newspapers, radio, and podcasts.
- MSN.com is a visited portal website provided by Microsoft.
We will investigate/interested in looking at their emotion/view about the brexit later, most influencial media Sentiment Analysis
II.2 What is the country of origin of these media?
- Only looking at the domains we could find that most domains are based in UK as we expected. Scrapping the web page to get the domain countries using beautiful soup for future analysis.
- Note that to decide to which country each domain is assigned, we used the link that can be not always reliable since some domains can use .com.
II.3 What media and websites tend to have positive or negative statements towards the situation?
We observe that, considering the top 20 media outlets present in Quotebank's data, most of them have a negative sentiment towards the Brexit, regardless of their views. This is noted particularly with the Daily Express (here express.co.uk) which is a very conservative tabloid in the UK. It is surprising to note that most of the opinions are negative despite the fact that the Brexit was voted for yes. Again, we must keep in mind that this does not reflect the opinion but rather the tone of the quote.
II.4 How sentiments of quotations revealed by top 10 medias change over recent years?
We can see that generally, the propostion between negative, positive and neutral feeling stayed consistant throughout the years. We can nonetheless notice again that the number of quotes in 2020 always drop.
III - Speaker views towards Brexit
III.1 : What are the keywords in Brexit-related quotations?
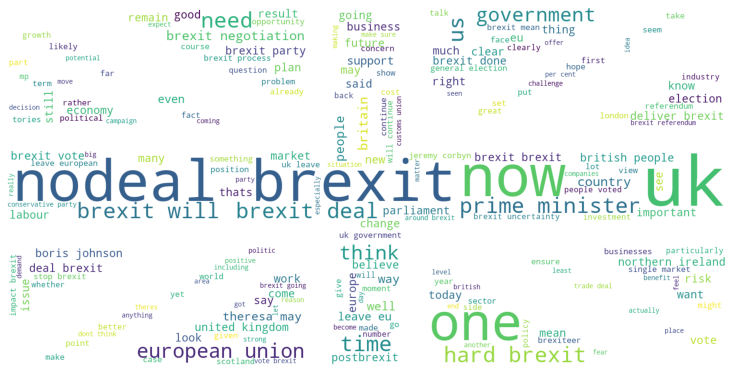
We see that Brexit and no deal are the most predominant words in the quote. Then comes prime minister, government, european union. We can also see some popular names such as Boris Johnson and Theresa May. Through this word cloud, we can actually say that the filtered quotes capture the topic of Brexit well and are consistent.
III.2 How many active speakers are mentioned?
We can see that the most popular speaker is Theresa May, former Prime Minister. This is not surprising knowing that she was in the midst of this whole Brexit issue and did all her companionship around it. Next is the current Prime Minister, Boris Johnson, of the Conservative Party, who was also involved in the Brexit issue. In 19th spot we find Donald Trump , who was the President of the USA for most of the time studied. For more details on the speakers, their parties and their views, please refer to our topic analysis (LDA).
III.3 How top 10 active speakers' sentiments changed over recent years?
We first notice the wide discrepancy between the amount of citations coming from the first two speakers compared to the remaining top 10 speakers. This difference must be even more drastic for the rest of the speakers. We can see that most of the quotes from Theresa May contain a positive sentiment towards the Brexit. While this may seem surprising, we see that Boris Johnson's quotes are mostly negative, but keep in mind that the sentiment analysis retained the overall sentiment of the quote, not the opinion on the Brexit.
III.4 What are the main topics discussed? (LDA analysis)
From the plot below we can notice the major topics keywords: We can see that some of them are projecting the Brexit as an opportunity for a better change, and some of them are reflecting all the risks that may occur, stating words like (risk, damage, lose, crisis, impact etc..) And also, we can see how some keywords are reflecting the will the stay in Europe (partners, stay, deal, relationship, members etc)
During the topic analysis with LDA, we gathered an idea of the key words and topics in trend. However, it would be interesting to go one step further and analyze the position of the most involved Brexit speakers. For this, we looked at the most active ones and managed to distinguish two different groups. On the one hand the predominant speakers who are in favor of Brexit are Boris Johnson, Nigel Farage and Matthew Elliott for instance, as they believe that Britain is better off outside the European Union. On the other hand, the speakers who are completely against Brexit like David Cameron, George Osborne, Enda Kenny and Christine Lagarde, were worried about the consequences of this move.
III.5 Where do they come from?
Again, we see that most of our speakers are from the UK, which is quite normal. Unlike the media, which was concentrated in Europe and the commonwealth countries, the second most represented nationality is American. Then we find the European countries and some commonwealth countries. One has also to note that the quotations have been extracted mostly from western media, thus the predominance of these regions in the statistics.
III.6 How has the number of active speakers changed over the different periods of Brexits?
Note how all the important dates, except for June 24, 2019, fall next to or exactly on a peak. We can also note that the shape of the quotation count time series looks very similar to the active speakers time series, but the scale is different. This is because we are filtering out unique speakers. Even though the two most active speakers, Theresa May and Boris Johnson, are heavily represented in the data set, they are not necessarily monopolizing the data as we can observe high and multiple peaks. Notice also that after the referendum on 2016, the number of active speakers peaked. Some weekend effects were also observed. Despite all this, there is no relevant trend related to important dates in the time series, the overall shape enters a stationary regime rather quickly.
III.7 What is their sentiment about the situation, content or not content, independently of their opinion on pro or against Brexit?
For the sentiment analysis, we used the results of three packages: Textblob, SentimentIntensityAnalyze and Flair. We have then taken the median of the three resulting polarities. To subsequently decide on the thresholds to choose for classifying the emotions (Positive, Negative, or Neutral), we manually labeled 100 quotes and selected the thresholds accordingly. It is critical to note that the resulting emotion does not reflect the direct view or perception of the Brexit, i.e. pro or con. The resulting emotion is based on the tone of the quote. As an example (fabricated), the two quotes: "Brexit is crucial, staying in the union would be a catastrophe, a suicide" and "Staying in the union is crucial, leaving would be a catastrophe, a suicide" will both be assigned a negative emotion by design even though they stand for two different opinions.
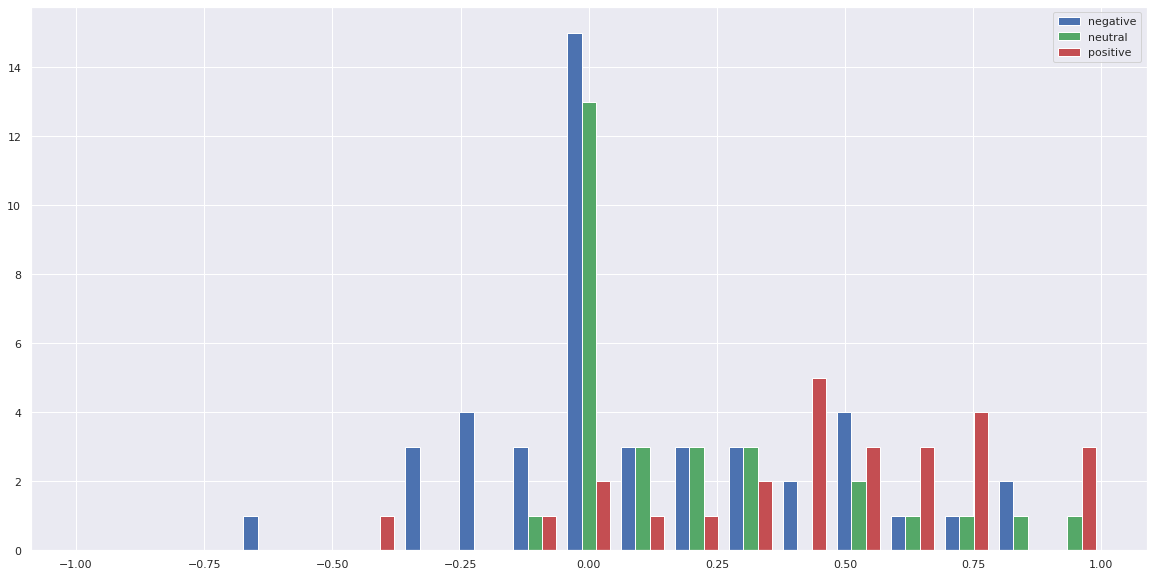
The above plot represents the distribution of polarities over the the three emotions for the manually labeled datapoints.
We can see that the choice of threshold is not necesserly easy. When manually labeling the data, we trying on capturing the tone and overall emotion portrayed by the message, not the opinion on itself. It was not always simple and the results are subjective. After looking at the histograms and trying multiple options, we ended up picking the following thresholds:
- polarities higher than 0.5 are considered positive.
- polarities lower than 0.2 are considered negative.
- the rest is neutral.
Positive quotes 27.45 %
Neutral quotes 24.26 %
Negative quotes 48.29 %
We can see that most of the quotations are negative. They are followed by positive and finally not to far behind by neutral quotations.
III.8 Did they change their perception during this period?
The distribution of the different emotions throughout time seem consistant, with the major part of the quotes being negative, and there's no sudden change in tone of the speech.
III.9 How are the different sentiments of the speakers distributed by countries?
Here we see how the two nationalities and the work profession seen/have different feelings towards Brexit. We can see that most professions have a more negative sentiment. Trade unionist, a left wing party mainly from the UK and Ireland, are strongly negative towards Brexit. For journalists, this is still the case, but the split is fairly even between the three emotions. We can also see that most of the people represented coming from the UK are politicians, while in other countries like the US, there are mostly journalists. In addition to journalists and politicians, we can observe that soccer players are very active. This may be due to the fact that the selected data filters out England words for example and that data may contain information about soccer.
Conclusion
The data with whom we have worked contains less than 0.08% of the total data in our quotation bank. The count of citations was very low prior to 2015 and began to increase through 2019 before dropping again. When comparing the number of citations to the important Brexit dates, no relevant trend emerged. Looking at the media aspect: most of the media come from the UK, and then from some Commonwealth countries. Most of them have negative emotions about Brexit, in consistent proportions over the years.
In terms of speakers, Theresa May and Boris Johnson are overwhelmingly the two most popular. In analyzing the speakers' views on the topic in more detail with the LDA, we made some important observations. Two main groups were noticed: the first where people project Brexit as an opportunity for better change, and the second where some of them reflect all the risks that can happen, using words like (risk, damage, loss, crisis, impact etc.). Our analysis also allowed us to see the position of the speakers most involved in Brexit in the three different timelines (before, during and after the referendum). The distribution of citations by speaker nationality confirmed our hypothesis: the countries with the most interest in the UK are the most involved in the Brexit situation.
In addition, we also found that most speakers are from the UK, followed by the US. Looking at the number of active speakers over time, we find that after the 2016 referendum, the number of active speakers peaked. In terms of their emotion, it remained constant over the year, no major changes were observed.
When focusing on their occupations, most occupations have a more negative sentiment. For journalists, the split is quite even between the three emotions. We can also see that most of the people represented in the UK are politicians, whereas in other countries like the US, there are mostly journalists.
The observations we have made so far are rather interesting but also a bit expected at some level. In the future we would like to look deeper into the data and work more on the interpretation of our Spectral Analysis. We would also like to do some regression to pinpoint the relationship between features and emotion. Finally, a good upgrade might be to consider Aspect Sentiment Analysis, either pre-trained on Brexit or trained it manually, so that emotion can be directly related to the speaker's opinion on Brexit.
Our Team
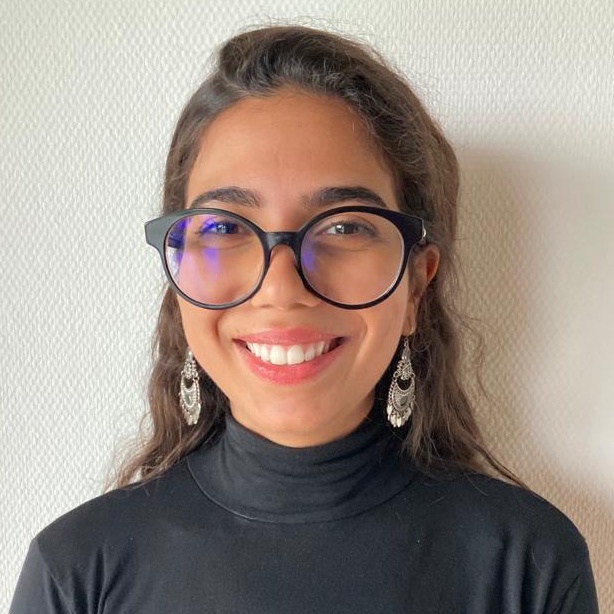
Zineb Agnaou
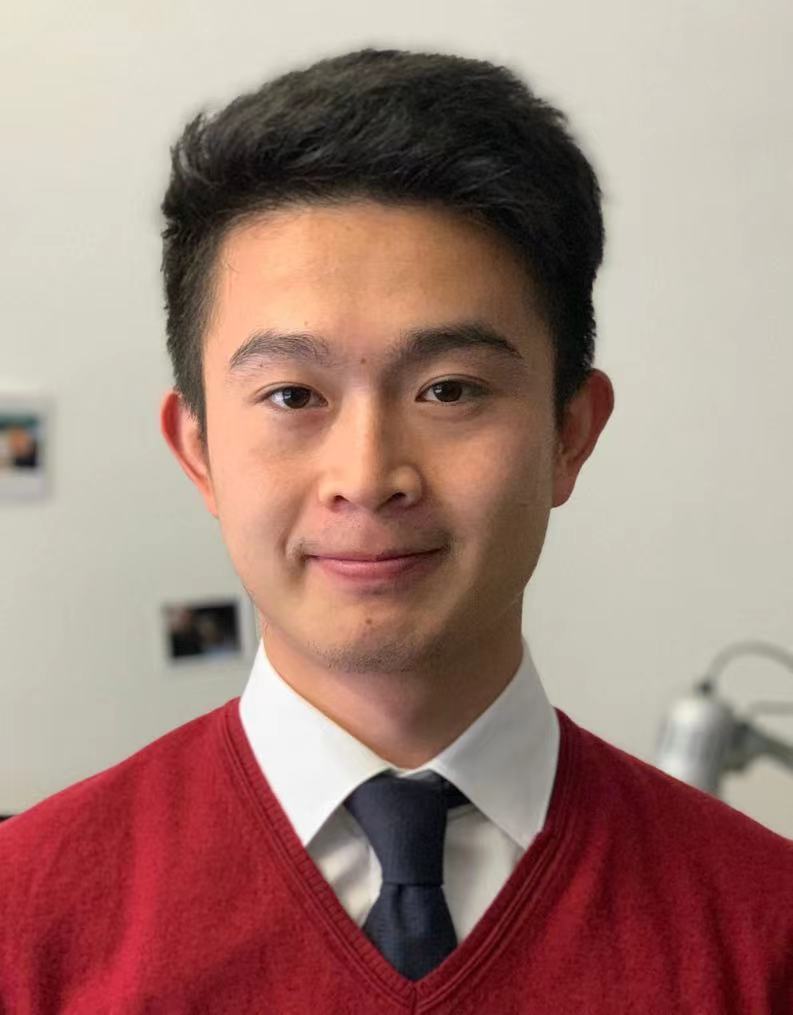
Jingrong Chen
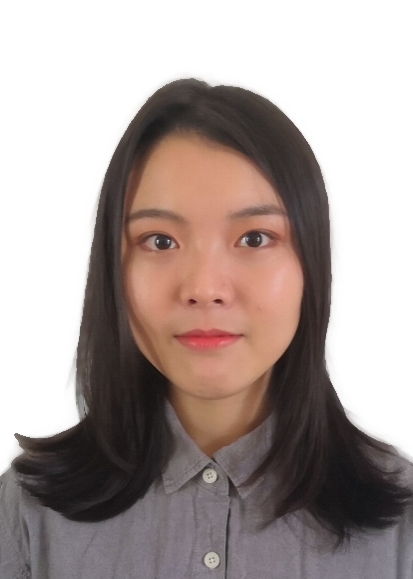
Haoyu Sheng
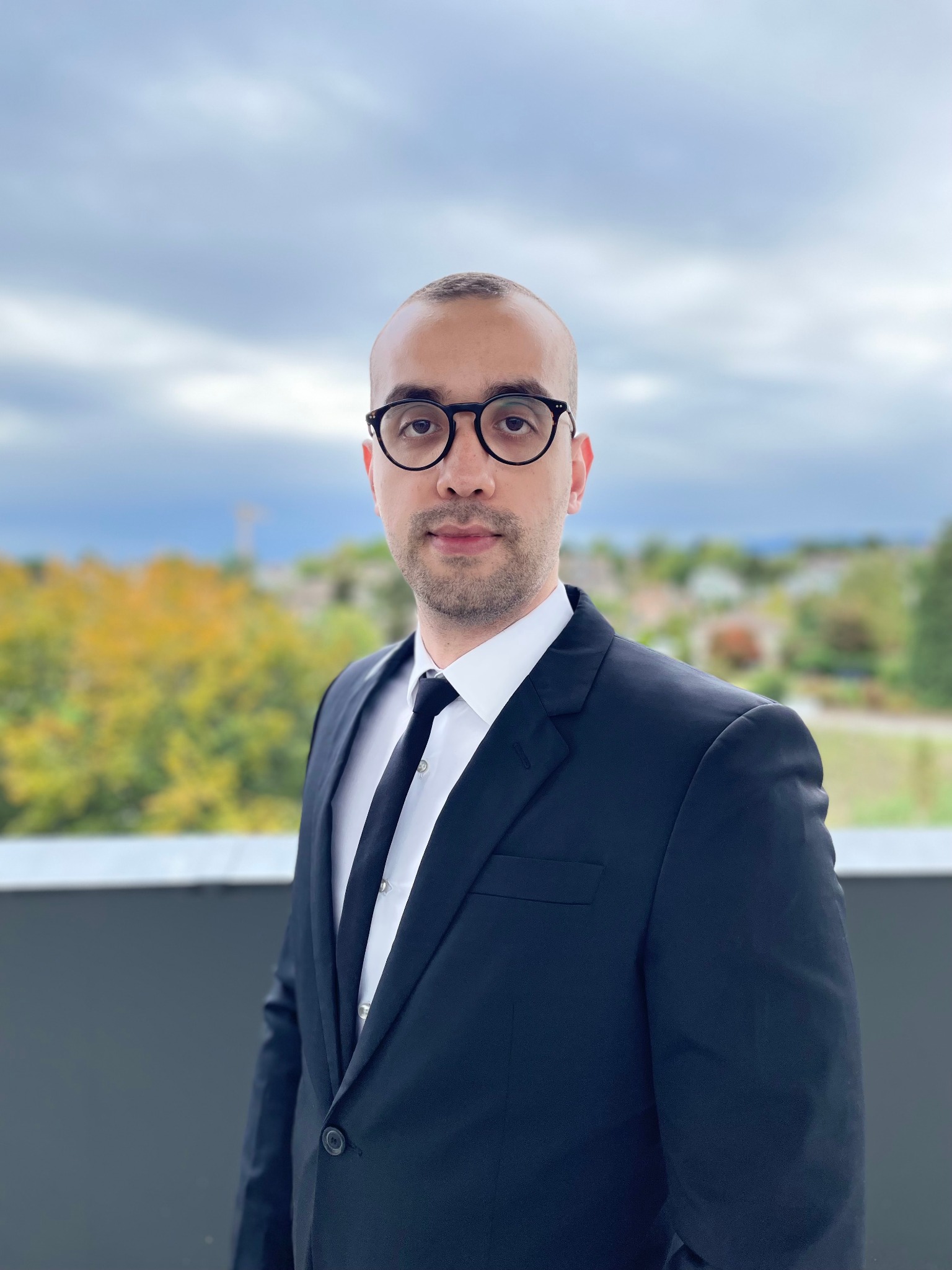